How Airtable Made Archive Validation Work at Petabyte Scale
They handled billions of rows using joins, hashes and a lot of buckets.
TL;DR
Situation
Airtable stores a huge amount of historical data (like undo logs) in sharded MySQL databases. This data rarely gets accessed but takes up nearly half their total storage, making it expensive to keep in hot storage.
Task
Move the old data to S3 buckets and validate that the archived version is identical to the original without hitting production systems or slowing anything down.
Action
Export MySQL snapshots to Parquet files in S3 using Amazon’s Relational Database Service (RDS) built-in export feature
Repartition and sort the data using Apache Flink and Kubernetes jobs to make it query-friendly
Load both the export and archive datasets into StarRocks for validation
Tune ingestion with bucketed partitions and shard-based parallelism to speed up loads
Use hash-based comparison and colocation joins in StarRocks to spot mismatches efficiently
Result
Airtable can now validate nearly a petabyte of archived data without touching live databases. The system runs fast, scales well, and made it safe to delete old data, cutting RDS storage costs significantly.
Use Cases
Data storage, archive validation, high-throughput ingestion
Tech Stack/Framework
MySQL, Amazon S3, Apache Flink, StarRocks, Apache Parquet, DynamoDB
Explained Further
Background: why this project happened
Airtable’s data lives in sharded MySQL (RDS) instances, with each customer base tied to a single shard. As bases grow, they accumulate append-only history data (like undo logs), which helps support features like revision history. This data isn’t accessed often, but over time it ballooned to nearly half of Airtable’s total storage footprint.
This wasn’t sustainable. So the Live Shard Data Archive (LSDA) project was kicked off to move that infrequently accessed data to cheaper storage (S3 buckets). Once safely archived, they could drop the data from RDS and reclaim space.
How the data was archived and prepped
The first step was exporting MySQL snapshot data to S3 in Parquet format. That was just the start. Because the data volume was huge—1PB+ across 10M+ files—they used Apache Flink to repartition the files by customer.
After repartitioning, a Kubernetes job handled sorting and indexing. Bloom filters were added to help the most common queries run faster once the data was live.
Early validation ideas (and why they didn’t work)
The goal of validation was simple: make sure the archived data was bit-for-bit identical to the original export. But with ~2 trillion rows and nearly 1PB of data, that wasn’t easy.
Comparing row-by-row against live RDS instances wasn’t an option cause it would’ve slammed production databases. So instead, they used the original Parquet exports as the source of truth, since they were also in S3.
Row-by-row comparison was still too slow even in S3. The better approach: load both datasets into a relational engine and run join-based comparisons. This led to the decision to use StarRocks.
Why StarRocks was a good fit
StarRocks was chosen because it handles large join operations more efficiently than other engines. The plan was to load both datasets (the RDS export and the S3 archive) into local StarRocks tables and run join comparisons between them.
StarRocks supports multiple input formats and storage options, but in this case, they pulled raw Parquet files into StarRocks’ local tables. This enabled colocation joins, a key feature that made row comparison at this scale possible.
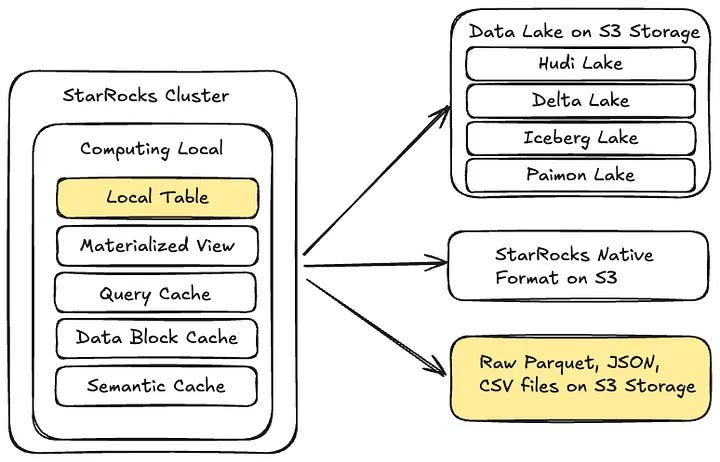
Getting ready to load at scale
Loading almost a trillion rows from hundreds of millions of small Parquet files into StarRocks is no small task. So they optimized for speed and throughput:
Reduced Replication: Set
replication_num
to 1, since this was a one-time job and didn’t need high availability.Increased Parallelism: Tuned StarRocks’ internal ingestion parameters (pipeline_dop, pipeline_sink_dop) to load more concurrently.
More Buckets: Limited each data bucket to 5GB max by increasing the number of buckets per partition. This avoided large compaction delays and boosted throughput.
These changes helped avoid a multi-month ingestion timeline and kept everything moving.
Ingesting the RDS export
They started with a basic table schema that included primary keys and a hash column of non-key fields for comparison. Ingestion was done via SQL insert statements that pulled from the Parquet files.
But they quickly ran into performance issues: loading two shards could take a full day, and parallelizing the ingest didn’t help much. Jobs would stall at 99% because StarRocks delayed finalizing the data (aka “taking effect”) after loading.
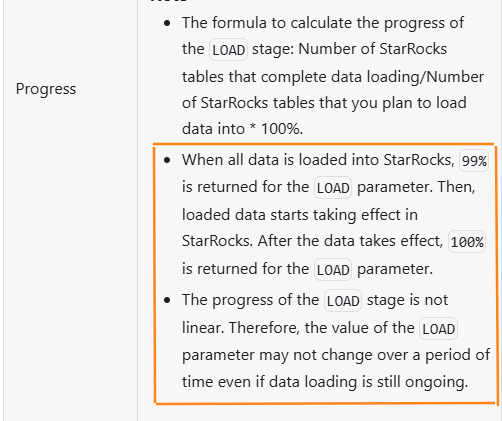
Fixing bottlenecks with buckets
Increasing the number of buckets was the first fix applied by the team. They went from no bucket definition to 7,200 for one of the smaller tables. This helped, but brought memory issues with it as some tablets exceeded memory limits for indexing large primary key sets.
Partitioning by shard for better distribution
Next, they partitioned the table by shard ID. This allowed each partition to have its own 64 buckets, spreading memory usage more evenly.
With ~148 shards, this gave them 9,472 total buckets, enough parallelism to load data efficiently without overloading memory. The result: full ingestion in under 10 hours, averaging around 2 billion rows per minute.
Loading the archive
With the source-of-truth (RDS export) ingested, it was time to load the archive data. It needed to follow the same partition and bucket setup to support efficient colocated joins in StarRocks.
They created a similar table for the archive, with shard ID as the partition key. But this data had a different storage layout in S3, which made ingestion harder.
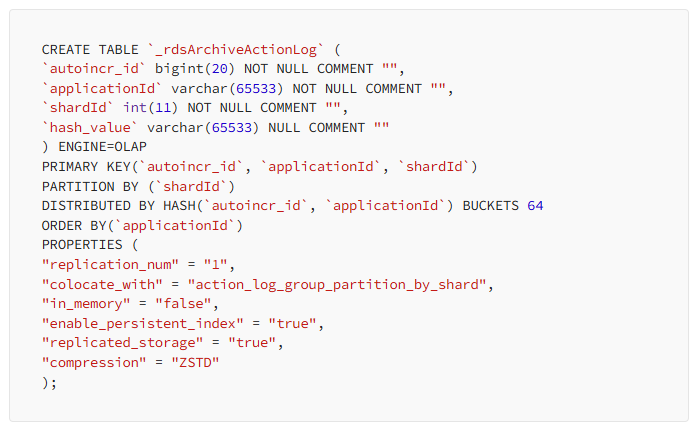
Discrepancies in directory structure
Unlike the export data (organized by shard), the archive was split into 6M+ small directories by application. And since the original shard ID wasn’t stored, they couldn’t extract it directly from the file path like they did with the export.
Instead, they relied on DynamoDB, which held file metadata. A Global Secondary Index on shard ID let them query which files belonged to each shard.
Dealing with millions of insert statements
Another issue: StarRocks only lets you specify one file path per insert. With 6M files, that would’ve meant 6M insert statements which was unmanageable.
They tried heavy parallelization but hit StarRocks’ versioning limits (1000 versions max per table). That caused insert jobs to fail under high concurrency.
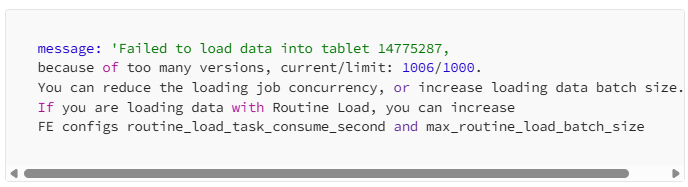
So they switched to batching inserts using UNION ALL
. For each shard, they grouped multiple files into a single insert like:
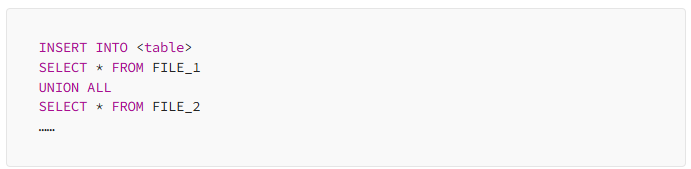
With this strategy, they were able to load data for 100 applications in parallel. That brought total load time for the archive side (~1T rows across 6M apps) down to just 3 days.
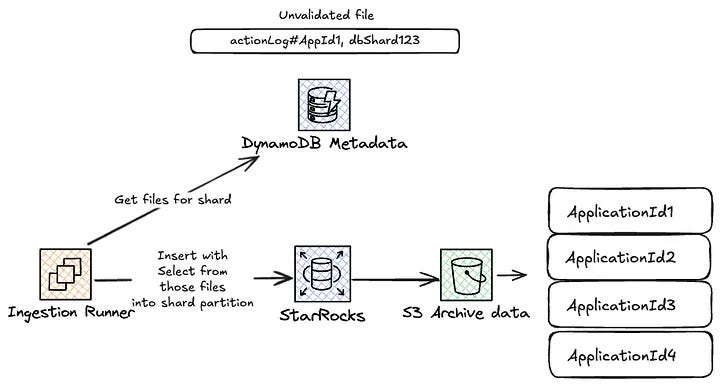
Results
By the end of the validation phase, Airtable had a working pipeline that could handle petabyte-scale data verification without touching production systems. Both the RDS export (source of truth) and the S3 archive were loaded into StarRocks, validated through colocation joins, and any discrepancies surfaced cleanly.
Performance-wise:
The final ingestion setup hit ~2 billion rows per minute
Archive ingestion (from 6M apps) completed in just 3 days
Export ingestion completed in under 10 hours
This validation work made it possible to safely delete nearly half of Airtable’s stored data from MySQL—cutting storage costs while still preserving query access through S3.
Lessons Learned
Don’t trust “just parallelize it”: Early attempts to boost ingestion throughput by running more jobs in parallel hit versioning limits and caused performance stalls. StarRocks’ concurrency limits required more careful batching and schema design.
Schema strategy matters: Partitioning by shard and tuning bucket counts was key to scaling ingestion. Trying to force everything through one large table without partitions caused memory issues and slowdowns.
Plan around your file layout: Export data was easy to ingest because it had shard structure in the directory path. Archive data didn’t which meant they had to build DynamoDB lookups to reconstruct that mapping. File structure affects more than storage; it affects ingestion and validation too.
UNION ALL is your friend (sometimes): Combining insert statements using
UNION ALL
turned out to be one of the simplest and most effective ways to batch small file loads without tripping over StarRocks’ version limits.Ingestion tuning pays off: Small changes like reducing replicas, tuning
pipeline_dop
, or setting 64 buckets per shard had a huge impact on total load time. The right combo of schema and cluster config made a 1PB job manageable.
The Full Scoop
To learn more about this, check Airtable's Engineering Blog post on this topic