No Cookies, No Problem: Grammarly’s Ad Experiment
How Grammarly Measured Ad Impact Without Tracking 30M+ Users
TL;DR
Situation
Grammarly’s 30M+ daily users rely on paid ads, but traditional attribution failed to measure YouTube’s true impact, especially with third-party cookies disappearing.
Task
Find a privacy-first way to determine if YouTube ads drive new users or just capture organic sign-ups using geo experimentation.
Action
Geo Experimentation: Stopped ads in select regions while maintaining them in others to measure impact.
Data Modeling: Used Google’s TBR package with a BSTS model to estimate incremental user acquisition.
Geo Split Selection: Created balanced test/control groups using clustering and randomization.
Power Analysis: Optimized experiment duration and sample size for accuracy and minimal business disruption.
KPI Measurement: Focused on new active users instead of revenue, aligning with Grammarly’s freemium model.
Result
YouTube ads were most effective before peak seasons but had less impact during them. When ads were paused, new sign-ups dropped immediately, showing YouTube’s stronger mid-funnel role than expected.
Use Cases
Marketing Attribution Improvement, Media Spend Optimization, Incrementality Measurement
Tech Stack/Framework
Google TBR (Time-Based Regression), Bayesian Structural Time Series (BSTS) Model, Clustering, Randomization
Explained Further
Understanding the Attribution Challenge
Measuring marketing effectiveness is challenging, especially for ads that don’t involve direct clicks. It’s easy to track whether someone clicks on a Google search ad, but what about an ad they only view on YouTube? Traditional attribution models often struggle to assign proper credit to top-of-funnel marketing, leading to an undervaluation of video ads and TV campaigns.
To grasp this issue, it helps to break down the most commonly used attribution models:
First-Touch Attribution assigns full credit to the first marketing interaction. If a user first sees a YouTube ad and later signs up via a search ad, YouTube receives all the credit—even if the search ad played a role in the final decision.
Last-Touch Attribution gives all credit to the final touchpoint before conversion. In the same example, search would receive full credit, completely ignoring YouTube’s contribution.
Multi-Touch Attribution distributes credit across multiple touchpoints along the user journey. While this approach is more sophisticated, it often relies on predefined weighting rather than causal analysis, making it difficult to assess the actual incremental impact of each channel.
These models work well for click-based channels like search, where direct interactions are recorded, but they fail to capture the influence of upper-funnel ads like YouTube.
The problem is further compounded by the deprecation of third-party cookies, which limits tracking across websites and devices. Without this capability, marketers struggle to evaluate how ad exposure influences user behavior. Instead of relying on outdated tracking methods, Grammarly’s data science team turned to geo experimentation, a method that measures ad impact without needing third-party tracking or user-level data.
Designing the Experiment
Geo experimentation functions like an A/B test but is conducted at the geographic level rather than the user level.
Defining Geographic Units: Users are segmented by Designated Market Areas (DMAs), which are self-contained media markets originally created for television advertising.
Turning Off Ads in Test Regions: In the test group, YouTube ads are paused, while in the control group, they continue as usual.
Measuring Incremental Lift: At the end of the test period, key performance indicators (KPIs) are compared between test and control groups to determine whether ad spend generated a statistically significant lift.
Although conceptually simple, executing a geo experiment requires rigorous planning. Selecting the right regions, ensuring test and control groups are balanced, and controlling for external factors such as seasonality are all crucial to obtaining reliable results.
Choosing the Right Locations
Selecting which locations belong in the test and control groups is one of the most critical steps in the experiment. Ideally, these groups should be as similar as possible before the test begins, ensuring that any observed differences are due to ad exposure rather than pre-existing market conditions.
With 210 DMAs in the U.S., there are more than 1,000 possible ways to split them. Grammarly’s team evaluated multiple approaches to ensure an unbiased and statistically sound selection process:
Clustering: DMAs with similar historical KPI performance were grouped before being randomly assigned to test and control groups.
Randomization: An alternative approach ignored pre-grouping and assigned DMAs randomly, allowing for a broader range of possible configurations.
To validate the quality of the split, the team assessed:
Root Mean Square Error (RMSE): Used to confirm that the historical performance of test and control groups was similar before the experiment began.
Predictive Stability: BSTS (Bayesian Structural Time Series) modeling was applied to test whether a synthetic control could accurately forecast performance in past data.
After testing multiple configurations, the most statistically balanced split was selected.
Ensuring Statistical Rigor with Power Analysis
A key consideration in designing the experiment was ensuring sufficient statistical power to detect meaningful changes.
Too few test locations could result in inconclusive findings.
Too many could increase costs by pausing ad spend in major markets unnecessarily.
To find the optimal balance, Grammarly’s team simulated different levels of ad impact (e.g., 1%, 2%, 3%) and assessed whether the experiment could reliably detect them. This process helped refine the test duration and sample size, ensuring clear, actionable results while minimizing business disruption.
Measuring the Impact of YouTube Ads
Since Grammarly operates on a freemium model, revenue isn’t the best immediate indicator of marketing effectiveness. Most users sign up for the free version first and upgrade later, making new active users a better KPI for assessing ad-driven growth.
To isolate the true impact of YouTube ads, Grammarly used causal inference techniques, specifically:
Bayesian Structural Time Series (BSTS) Modeling to create a counterfactual baseline, predicting what would have happened if YouTube ads had remained active.
Comparing Actual vs. Predicted Performance to determine whether new sign-ups declined only in test regions, indicating the ads’ incremental effect.
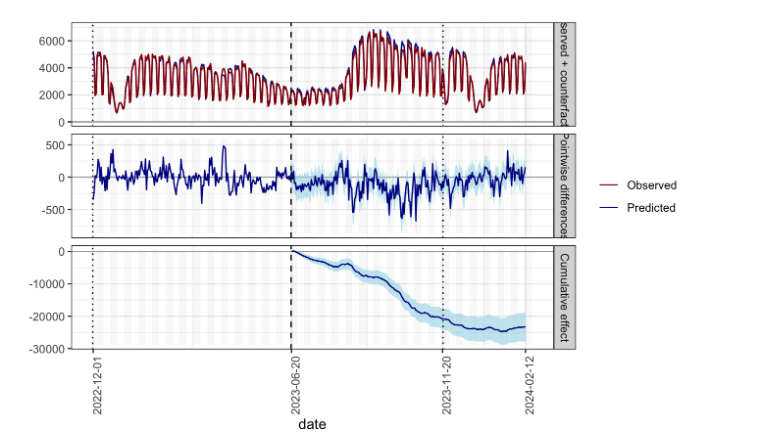
By applying this approach, Grammarly was able to separate the impact of YouTube ads from seasonal trends and other external variables, providing a more accurate measurement of ad effectiveness than traditional attribution models.
Results
The experiment provided several insights into how YouTube ads influence user acquisition.
Timing Has a Major Impact
YouTube ads had a stronger effect before Grammarly’s seasonal peak but were less effective during peak months when organic sign-ups naturally increased.
These findings suggest that shifting ad spend to pre-peak periods could generate higher returns than increasing spend during peak months.
Cost Efficiency is Best Measured Incrementally
Instead of tracking cost per new user, Grammarly now focuses on incremental cost per new user, which accounts for baseline conversions that would have occurred even without ads.
This refined metric provides a clearer picture of ad efficiency, allowing for smarter budget allocation.
YouTube Ads Work Faster Than Expected
The expectation was that video ads would take time to influence conversions. However, the test revealed an immediate drop in new sign-ups after ads were paused, suggesting that YouTube plays a more direct role in driving conversions than previously assumed.
Lessons Learned
Timing Ad Spend for Maximum Impact – Ads were more effective before peak seasons than during them. Shifting budgets to pre-peak periods can improve ROI.
Rethinking Cost Efficiency – Tracking incremental cost per new user provides a clearer picture of true ad impact, avoiding the pitfalls of standard cost metrics.
YouTube’s Role in the Funnel – Video ads drove faster conversions than expected, suggesting they play a mid-funnel role rather than just top-of-funnel awareness.
Geo Experimentation as a Scalable Alternative – As privacy restrictions grow, geo experiments offer a reliable way to measure ad effectiveness without user tracking.
Experiment Design is Key – A well-planned test, with proper geo splits, power analysis, and robust modeling, ensures meaningful and actionable results.
The Full Scoop
To learn more about the implementation, check Grammarly Blog post on this topic