Scaling Apache Flink: How Reddit Cut Memory Usage by 60%
Optimizing real-time ad validation with field filtering, tiered storage, and infrastructure enhancements.
Situation
Reddit's advertising platform processes thousands of ad engagement events per second, necessitating real-time validation and enrichment to ensure accurate reporting and prevent budget overdelivery.
Task
Develop a scalable, real-time ad event validation system capable of efficiently handling high event volumes while maintaining performance and reliability.
Action
The engineering team developed the Ad Events Validator (AEV) utilizing Apache Flink to correlate ad server events with user engagement events. To overcome issues related to large state sizes and resource demands, they implemented:
Field Filtering: Conducted a thorough analysis of downstream data consumption, establishing an allowlist that significantly reduced the event payload size by 90%, leading to CPU and memory usage reductions of 25% and 60%, respectively.
Tiered State Storage: Integrated Apache Cassandra for external state storage, effectively reducing in-memory state size and enhancing the efficiency of checkpointing and system recovery processes.
Result
These strategic enhancements resulted in a more scalable and cost-efficient AEV system, improving overall performance and operational effectiveness.
Use Cases
Real-Time Event Validation, Data Enrichment, Resource Optimization
Tech Stack/Framework
Apache Flink, Apache Kafka, Apache Cassandra
Explained Further
Background
Reddit processes thousands of ad engagement events per second. These events require validation and enrichment before being sent to downstream systems. Key components of this validation process include applying a standardized look-back window and filtering out suspected invalid traffic.
In addition to a batch validation pipeline, a near real-time pipeline improves budget spend accuracy and provides advertisers with real-time insights into campaign performance. This real-time component, known as the Ad Events Validator (AEV), is built using Apache Flink. AEV matches ad server events with engagement events and writes the validated results to a separate Kafka topic for downstream consumption.
Building and maintaining AEV though, presented several challenges to the Reddit team
1st Challenge: Addressing High State Size Issues
AEV operates by matching ad engagement events with ad server events within a predefined look-back window. Each validated event consists of fields from both the ad served event and the engagement event. Because engagement events can occur at any point within the look-back window, ad served events must be stored in Flink state to ensure availability.
Scaling Issues
As Reddit’s advertising system evolved, the payload size of ad served events increased due to additional metadata. Combined with increasing event volume, this led to significant growth in Flink state size, introducing several challenges:
Slow Checkpointing & Timeouts – Checkpoints started taking up to 15 minutes, breaching SLAs.
Slow Recovery from Restarts – Large state snapshots stored in S3 took several minutes to load.
Limited Scalability – As event traffic increased, horizontal scaling options became exhausted.
High Cost – The Flink job required hundreds of CPUs and terabytes of memory.
To address these issues, field filtering was implemented to reduce payload size and tiered state storage was introduced to optimize local Flink state usage.
Optimizing with Field Filtering
AEV was initially designed to replicate the batch validation pipeline in real-time, maintaining consistency across both systems. Over time, an analysis of downstream usage revealed that most fields in the ad served payload were unused, including some of the largest ones.
Key Design Choices
Targeted filtering using an allowlist was chosen over a denylist to ensure resource efficiency.
This approach significantly reduced event size by 90%, leading to 25% lower CPU usage and over 60% memory savings.
The tradeoff: adding new fields requires a deployment, but the rate of field additions has been low, making this manageable.
Introducing Tiered State Storage with Apache Cassandra
Before implementing field filtering, an exploration was conducted into offloading state storage to an external system. Analyzing event patterns revealed that most engagement events occur shortly after an ad is served, making it possible to optimize how events were stored.
Key Optimizations
Local State for Recent Events – Ad served events were stored in Flink state only for the initial portion of the look-back window.
External Storage for Older Events – Events beyond this period were moved to Apache Cassandra, reducing Flink’s in-memory state size.
Custom State Lifecycle with KeyedCoProcessFunction – Event time timers were used to manage when to retain or offload ad served events.
These changes led to faster checkpointing, lower memory usage, and improved system resilience.
Challenge 2: Infrastructure Sensitivity and Maintenance
AEV's performance was impacted by Kubernetes cluster maintenance, including rolling restarts and version upgrades, leading to increased processing latency.
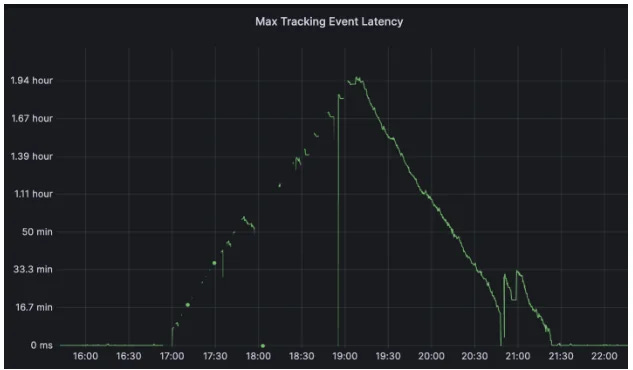
Issues Observed
Task managers taking longer to recover increased processing delay.
Kubernetes marked pods as “ready” before they were fully operational, causing inconsistencies.
Pod terminations disrupted ongoing checkpoints, further slowing recovery.
Optimizations Implemented
Flink Configuration Adjustments:
Configured
slotmanager.redundant-taskmanager-num
to provision extra task managers.Enabled
state.backend.local-recovery
for faster restarts by leveraging local state files.
Kubernetes Lifecycle Improvements:
PreStop Hook – Delays termination until ongoing checkpoints complete.
Startup Probe – Ensures pods are marked “ready” only after registering with the job manager and completing an initial checkpoint.
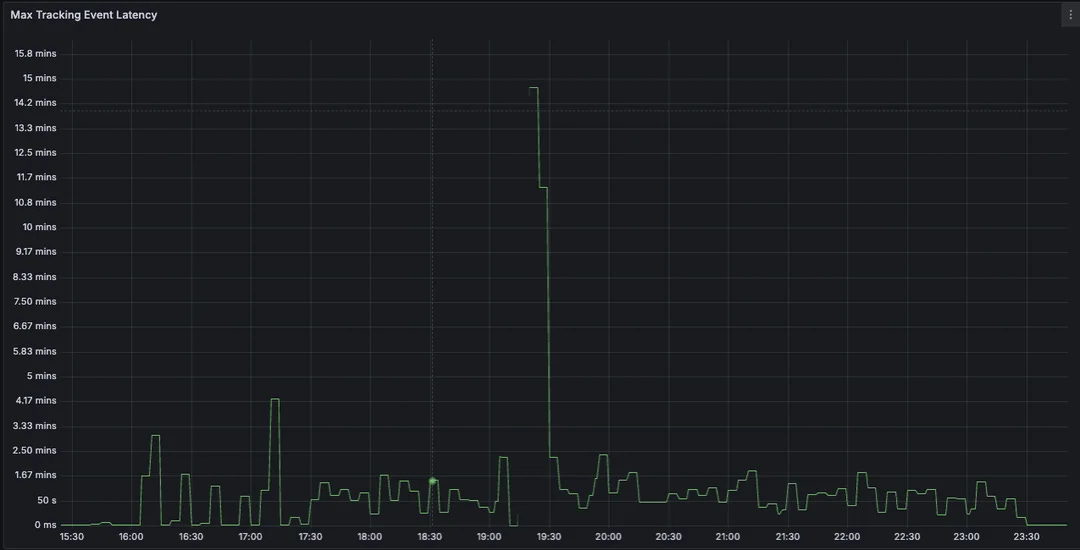
These improvements stabilized processing during maintenance, reducing latency spikes and improving system reliability.
Results
The implementation of field filtering and tiered state storage significantly improved the performance and efficiency of the Ad Events Validator (AEV). These optimizations led to:
Memory and CPU Savings: A 90% reduction in event size resulted in a 25% decrease in CPU usage and over 60% reduction in memory consumption.
Improved Checkpointing and Recovery: The use of Apache Cassandra for external state storage reduced the in-memory state size, leading to faster checkpointing and recovery times.
Increased Scalability: The tiered storage solution allowed AEV to handle higher event volumes without reaching the horizontal scaling limit.
Operational Cost Reduction: Resource allocation optimizations contributed to significant cost savings while maintaining system performance.
Lessons Learned
Several key insights emerged from the optimization of AEV:
Proactive Analysis of Downstream Data Usage: Identifying unnecessary fields early in the pipeline can yield substantial resource savings.
Tiered Storage Provides Flexibility: Using a hybrid approach of local and external storage allows systems to balance performance and cost efficiently.
Infrastructure Maintenance Requires Strategic Planning: Proper configuration of Kubernetes lifecycle policies can greatly reduce the impact of pod terminations and rolling restarts.
Asynchronous State Retrieval is Effective: Offloading older events to an external store while processing recent events locally maintains both speed and efficiency.
The Full Scoop
To learn more about this, check Reddit's Engineering Blog post on this topic